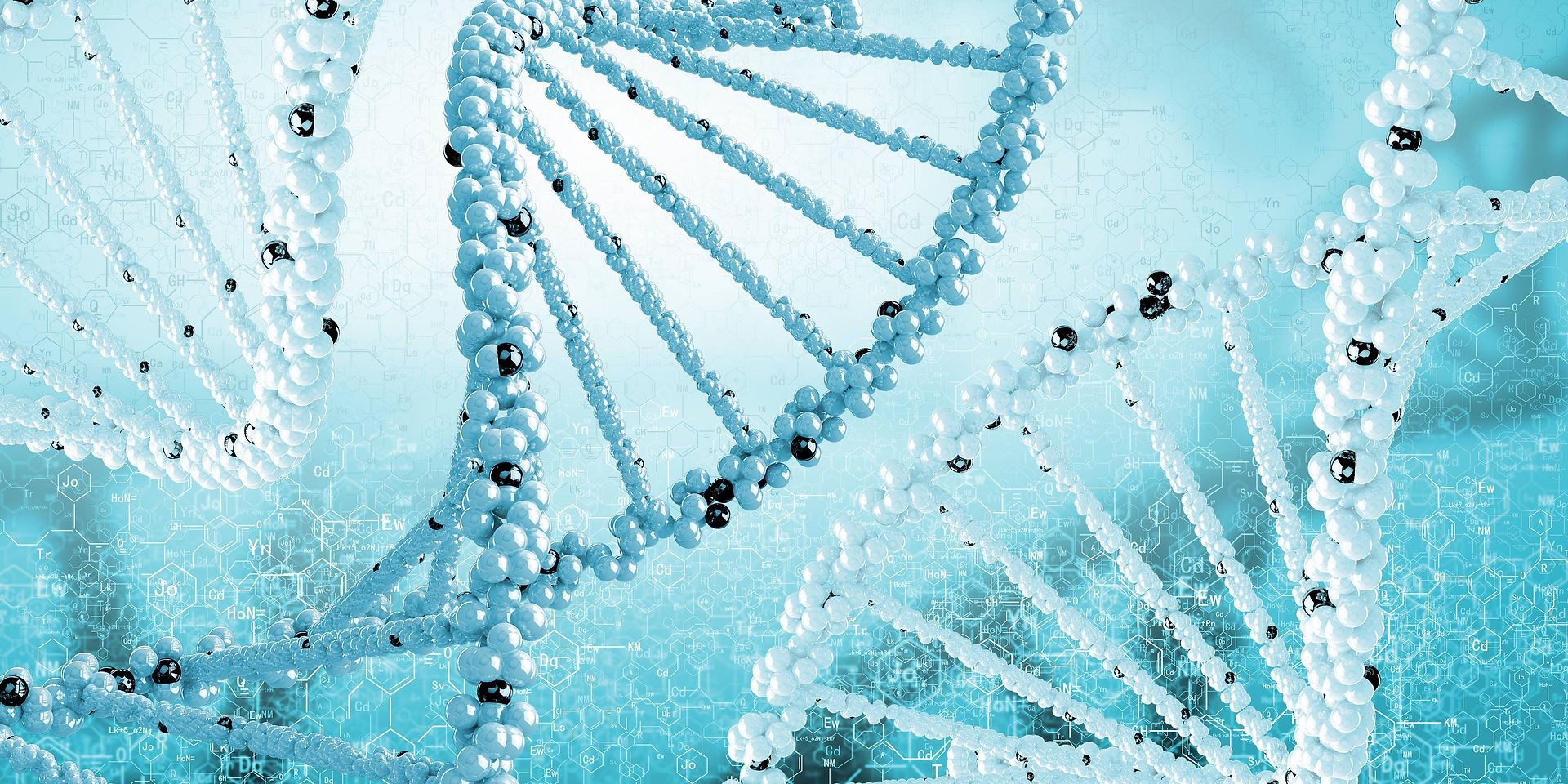

Medicine
The routine discovery of novel techniques of sequencing and the ever changing field of genomics provides us with a lens to visualize a future greater than ever. Ever since the discovery of the structure of the DNA, scientists have paved their way through years of hard work and research in order to bring us to the point where personalized medicine looks possible in the future. Personalized medicine is the process of analyzing a person’s genes in order to figure out the exact mutations and to provide the patient with medication that is fit to their symptoms and illness alone so that the treatment process is as efficient as possible. The journey has to this point been long enough to help lower the cost of sequencing per base pair. Imagine how prevalent it is to our future!
​
​In DNA sequencing, data generation is the foremost and the most essential step to any clinical procedure. For any analysis to be made, it is essential that data is extracted from the several sequencing platforms. To be most efficient, it is important to perform the following set of procedures in order to gain valuable information: 1) aligning the sequence to a reference genome, 2) base calling (alignment of bases to chromatogram peaks) 3) and the identification of the sequences through comparison with the reference genome. The amount of bases that are correctly aligned is called the depth of coverage and researchers have a benchmark which is a 30-fold (30x) average depth of coverage to know if a genome sequence is of high quality.
The Human Reference Genome and Its Limitations
Earlier, there is a mention of a reference genome and you must be wondering what exactly that is? The reference genome is a digital set of DNA sequence database which belongs to a specific species with their genes allotted to it. The reference genome mentioned here is a result of sequencing done through the help of anonymous donors. It is the most accurate and it covers 99% of chromosome readings. The limitation is set by the amount of samples used which makes the variations in these genes extremely small. By performing several tests, the NCBI lab deduced that in every 1.6 million genomic positions, around 800,000 positions differ from the reference genome. Moreover, several alleles in the reference genome includes both common and rare disease alleles. For instance, there are more than 20 rare disease alleles such as the Factor V Leiden allele which causes hereditary thrombophilia (increased blood clott). A potential solution to this problem is using the most common alleles in the reference genome.
Aligning Sequence Reads to the Reference Genome
Prior to the novel technologies of today, the only way of aligning sequences was through the algorithm, mapping alignment with quality (MAQ). However, this procedure turned out to be too superior for its use with longer sequences. Algorithms as such have the capacity to be run on simple things such as high-memory core desktops and laptops, parallel computing platforms and much more to run sequences but only a few companies/labs allow such access. A more appealing approach is to take several of these labs together and to perform an on demand parallel computing where the sources are shared and done simultaneously.
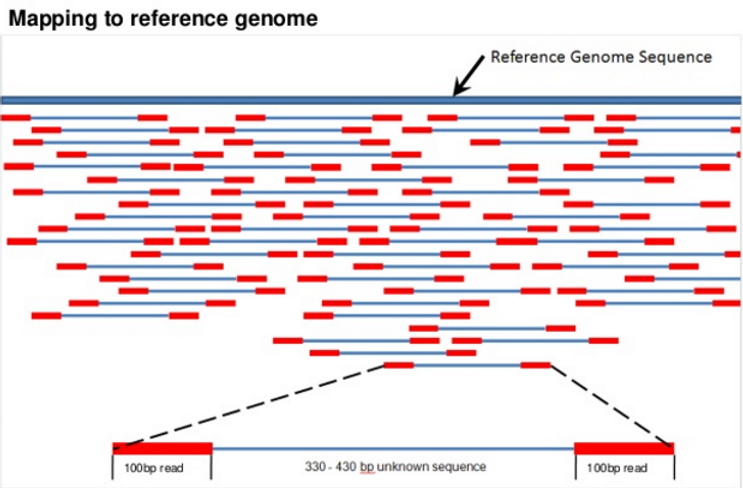
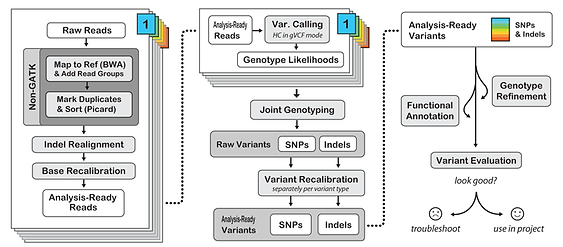
Identifying Single Nucleotide Variants and Small Insertions/Deletions
After the alignment process, sequences are read at the genomic positions in order to give it specific base calls so that it is divided based on certain characteristics. Using algorithms, these sequences are sorted based on the quality of reading and the depth of coverage. Several of the sequences are further narrowed down to bases that contribute to 16 genotypes at a single position. Taking these genotypes, a single genotype is chosen that matches significantly to the original reference genome with only a slight difference in one of its bases.
This single piece is then kept for further analysis. Although seemingly complex, you can think of this process like a series of dilutions. Each time, the sequences are diluted down from thousands to only a few in order to ultimately match it to the reference genome. This process has some important ramifications. First, since the reference genome is necessary for figuring out genetic variations between two sequences, certain diseases can be overlooked in the process. Since the haploid pairing of these sequences have similar alleles, no variants will be found. Next, the overlapping of sequencing can cause some variants to be overlooked yet again. However, there is a method to solve this issue. SAMtools and Genome Analysis Toolkit help to identify variations in these sequences which have base calling algorithms as well. Finally, since the reference genome that we have today is a combination of the sequences of a significantly small ethnic group, there is yet efforts to be made that would incorporate all of the sequences of every human race in the world. This impedes the alignment of certain bases that might indeed be a part of the reference genome and therefore, certain variations are never even found.
Identifying Large Structural Variants
Identifying larger variants in the sequencing procedure was a very common thing to ignore in the first generations of DNA sequencing; however, it turns out that these larger variants are essential due to their connection to diseases such as Mendelian and other complex diseases. These diseases include Familial Dilated Cardiomyopathy, Autism Spectrum Disorders, Idiopathic Mental Disorder, Schizophrenia, and Crohn’s disease. Since these variants account for more than 15% of the identification of diseases in sequencing, there have been many methods developed to help identify them. These methods include mate pair sequencing, regional variation in read depth, and split-read mapping. The first method helps with short reads where two ends of a DNA molecule is sequenced and is separated by base pairs. An alternate yet similar method is called the paired end sequencing where sequences are taken from the ends of an amplified DNA molecule. A reference genome is used again to compare the medium insert size of the reads to chromosomes contained within the reference genome. In the second method, there has to be significant differences between the read depth of the sequences which verifies for the presence of a rather large variant. This method works best for large inserts and deletions in the DNA fragments but most of the time, it cannot resolve upon these breaks in the DNA. In the final method, inserts are used to mark certain points on the reference genome or the sequence being interpreted as one side is used as an anchor while the other end looks for breaks in the DNA fragment. If there are any potential candidates found, then they are compared to known variants in order to make sure there were no false results.
Since these variants are the most helpful in letting us know about the potential disease that a patient may be carrying, it is important to reduce the amount of errors made during sequencing methods. One way to reduce errors is to use sequencing quality metric which increases the accuracy of the process. Also, using genotypes that include information about a whole family generation is more reliable than per single person. Despite these improvements, we are yet not in a position where we can successfully allow this method to be used in the medical field. For most cases utilizing this specific method, there is a need for a re-sequencing process using the Sanger method which is both time and resource extensive. This is the biggest barrier that will prevent bringing DNA sequencing into clinical trials until we work to improve upon the accuracy of data so that it does not have to go under re-confirmation after the first attempt at sequencing.
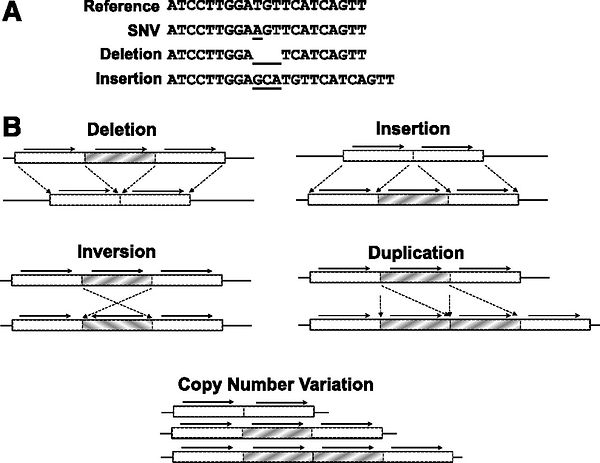
Parallel Sequencing:
Haplotype Phasing
A haplotype (set of genes in an organism inherited from a single parent) phase is most essential for understanding sequences containing chromosomes with diseases, the genotype and phenotype connections of compound heterozygous and oligogenic risk alleles, and the consequences of genetic variations. A haplotype phasing itself is a count of the amount of haplotypes in a given genotype data. This technology becomes essential to use when there is an abundance of sequence data that lack a genotype estimation when it undergoes procedures like chip based genotyping. Since haplotype phasing requires a long set of data, it isn't much useful when short reads are employed. Short reads can be used however, with algorithms based on pedigrees, with combined short reads and common population haplotypes for high throughput sequence data. Further improvement in this area will provide us with the chance to investigate disease biology.
High Throughput Sequencing and Mendelian Genetics
Using next generation sequencing methods, cardiovascular diseases have been helpful in the identification of mutations in genes such as BAG3 which causes dilated cardiomyopathy, familial aortic aneurysms caused by mutations in SMAD3, as well as mutations in AARS2 and ACAD9 in familial mitochondrial cardiomyopathy. Findings of these studies has paved a path for scientists to discover more about the human cardiovascular disease. Genes that do not contain diseases are sequenced through whole-genome sequencing and exome sequencing. To show how important this discovery is, let’s take a look at one example. While performing an exome sequencing method, a scientist identified the mutation SLC26A3 and hypothesized that it was caused by renal, salt-wasting Bartter Syndrome which allowed them to discover congenital chloride diarrhea. This allowed them to refine the steps that are taken for clinical treatment. Just imagine! The more that we are able to discover regarding diseases, the more closer we get to really refining certain treatments that are offered today so that it gives us better results for the future! Another example also includes the usage of exome sequencing. A mutation in the gene, XIAP was found in a patient with intractable Crohn’s-like inflammatory bowel disease. There was a diagnosis of of X-linked inhibitor of apoptosis (XIAP) deficiency which was followed up by a stem cell transplant with abiding improvements in the patient’s health.
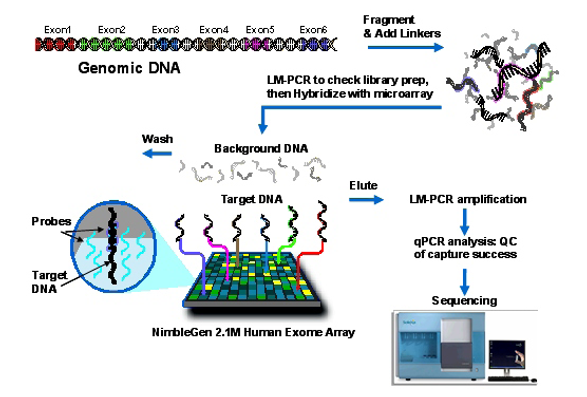
Exome Sequencing
Genome Sequencing and the Clinic
What makes Next Generation Sequencing so difficult for analysis is the fact that reference genomes do not include all of the information needed to exactly match with every single fragment of DNA sequenced. Possible solutions include the combination of clinical assessments and whole genome sequencing. This approach has also been used to detect severe disease recessive alleles since birth along with fetal aneuploidy by sequencing maternal blood samples. As aforementioned, not all genetic information are contained within the reference genome and therefore, there are external sources for extending this process and making the results more definite. Some of the annotation resources include the consensus coding sequence (CCDS) database, RefSeq, the UCSC KnownGenes database, and the GENCODE and ENSEMBL databases.
Other applications include the sequencing of RNA molecules thus allowing for the analysis of allele-specific expression, alternative splicing, and gene expression through the quantification of the RNA copy number. Using RNA is more efficient as well because it provides a greater accuracy and accurate gene expression. Another approach to take here is to merge high throughput sequencing with oligonucleotide sequencing for gene findings and mapping of genomic regions in order to detect diseases that are inherited. Another possible method is to use cell free DNA from the bloodstream and to find transplant DNA. This can greatly help patients with a need for endomyocardial biopsy surveillance.
Citations 4.1 - 4.6
Exploring the Depths of Life
​
Sequencing
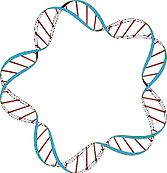